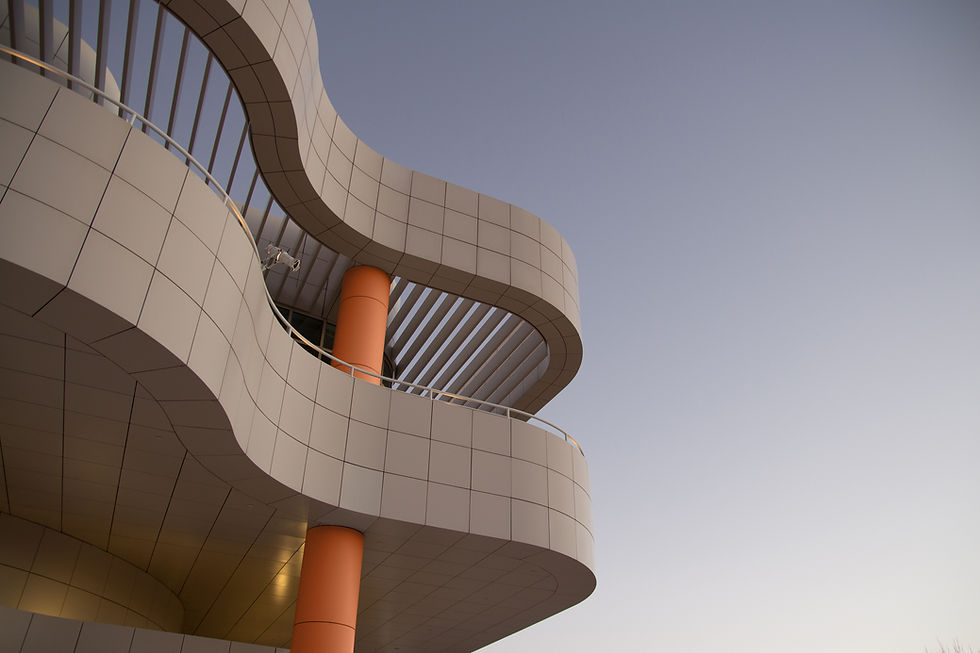
A Machine Learning Engineer's Guide to the EU AI Act
Jun 15, 2023
5 min read
0
82
0

This article was originally published on Forbes. Click here for the original version.
On Wednesday June 14th, the European Parliament passed the EU Artificial Intelligence Act (AI Act). The EU Parliament, Commission and Council will now engage in a final trialog process to negotiate the final version of the law. This process will likely take a few months before the act officially becomes law. Much like how GDPR caused a global paradigm shift for data privacy, the AI Act will have a significant impact in the way organizations develop, deploy, and maintain their AI systems, while also paving the way for further regulatory action from other legislative bodies around the globe.
Machine learning engineers should not be expected to understand the full nuances of the regulation. That will be the responsibility of legal or compliance teams. AI/ML practitioners should be focused on innovating and driving new opportunities for efficiency and effectiveness. However, new responsibilities will emerge for these teams so a basic understanding of the regulatory requirements will be necessary.
Let’s start with one point of clarification about the AI Act: the regulation does not directly target the models themselves, as it’s impossible for regulations to keep up with the pace of AI research & development and model versioning. Instead, they will seek to regulate the people and the processes for how organizations develop and deploy their AI use cases. This means that the ‘day to day’ of machine learning and data science practitioners is about to go through a radical shift to ensure AI use cases are properly documented, reviewed, and monitored.
Enactment of the EU AI Act is anticipated for early 2024 and full enforcement in 2026. Here are some key things AI/ML teams should know about this new regulated future.
Standardized Documentation
The AI Act classifies specific use cases, not ML models, into one of 4 risk categories: unacceptable (prohibited), high, medium and low risk. The act lists some specific uses of AI, such as ‘social score’ as prohibitively risky and outright bans them. High risk AI use cases are those used for purposes where malfunction or bias could result in severe physical, financial or emotional harm to an individual. This level of risk will come with some heavy regulatory requirements including registering it with an EU oversight agency. Medium risk systems are those where the user may be interacting with an AI agent and the act will require clear disclosure of AI to the user. Most other use cases of AI, except those covered by other existing regulations, will fall into the ‘low risk’ category where the law doesn’t impose a heavy regulator burden but does require an inventory of such use cases. AI/ML teams will be required to have their AI use cases documented and reviewed by a dedicated compliance or AI governance team to ensure the correct risk category is identified.
Documentation Accessibility
The sphere of stakeholders involved in AI Governance will expand, necessitating a shift in the way AI systems and use cases are documented. The traditional means of documentation, including code repository README files, system diagrams, code comments, and technical notebook outputs, will prove insufficient and inaccessible for non-technical users. New systems for documentation will emerge, and these solutions must effectively bridge the gap between technical intricacies, business-level concepts, and requirements, ultimately providing a comprehensive understanding of the technical work and its limitations within the broader context of the organization.
Generative AI Liability
One of the biggest changes the EU Parliament introduced in their version of the AI Act were clearer requirements for organizations deploying foundational models and generative AI systems. The exact set of requirements will be one of the biggest unknowns for the next few months, but starting to implement some testing and evaluation processes for generative AI use cases will be the best way to prepare. For example, conducting a small internal study on how often a given prompt may ‘hallucinate’, and documenting the results is a useful first step towards the kinds of risk mitigation efforts that the AI Act may require.
Testing & Human Evaluations
Many models are often evaluated by some error metrics derived from a held out set of training data. These are useful for training goals, but less so as a proxy for how well the model may perform in the real world. While many standard software systems have unit, integration and regression tests as part of a continuous deployment system, far fewer ML systems have these testing standards. Organizations should develop their own evaluation tasks that can be integrated into a testing suite to ensure model quality. In addition, having a standard set of evaluation tasks done by someone who wasn’t the model developer allows for an internal control that cannot be easily ‘gamed’.
Model Update Workflows
Any AI used for a ‘high risk’ use case will need to have structured processes defined for updating it. Different kinds of changes to an ML model may trigger a different level of review for compliance purposes. For example, minor adjustments to hyper-parameters might be deemed safe, while expanding the training dataset by more than 50% could raise compliance concerns. While the EU Commission has assured forthcoming guidance following the AI Act's enactment, organizations should proactively develop a comprehensive 'playbook' outlining which types of system changes necessitate different re-evaluation workflows and processes.
Conformity Assessment for High Risk Use Cases
Machine learning engineers need to be aware of the key aspects surrounding conformity assessments to navigate the regulatory landscape effectively. For internal assessments, organizations and service providers must establish a robust quality management system encompassing various elements, such as comprehensive risk management, diligent post-market monitoring, efficient incident reporting procedures (including data breaches and system malfunctions), and the ability to identify risks that were previously unknown. Additionally, stringent testing and validation procedures for data management should be in place. In certain cases, independent third-party assessments may be necessary to obtain a certification that verifies the AI system's compliance with regulatory standards – similar to other industries such as medical devices or food products.
The AI Act represents a landmark development in the regulatory landscape for AI, signaling significant changes ahead for machine learning engineers. While there are still uncertainties surrounding the final version of the AI Act, it is crucial for AI engineers and organizations to proactively prepare for the impending regulatory requirements. By staying informed, engaging in industry discussions, and collaborating with legal and compliance experts, AI engineers can navigate the evolving landscape with greater clarity and adaptability. Embracing responsible AI governance practices, implementing robust conformity assessment processes, and fostering a culture of transparency and accountability will be essential in ensuring effective compliance with the AI Act and building trust in AI systems. As the regulatory framework continues to evolve, the AI engineers will play a critical role in shaping a future where AI is deployed responsibly, ethically, and with the utmost consideration for the broader societal impact.