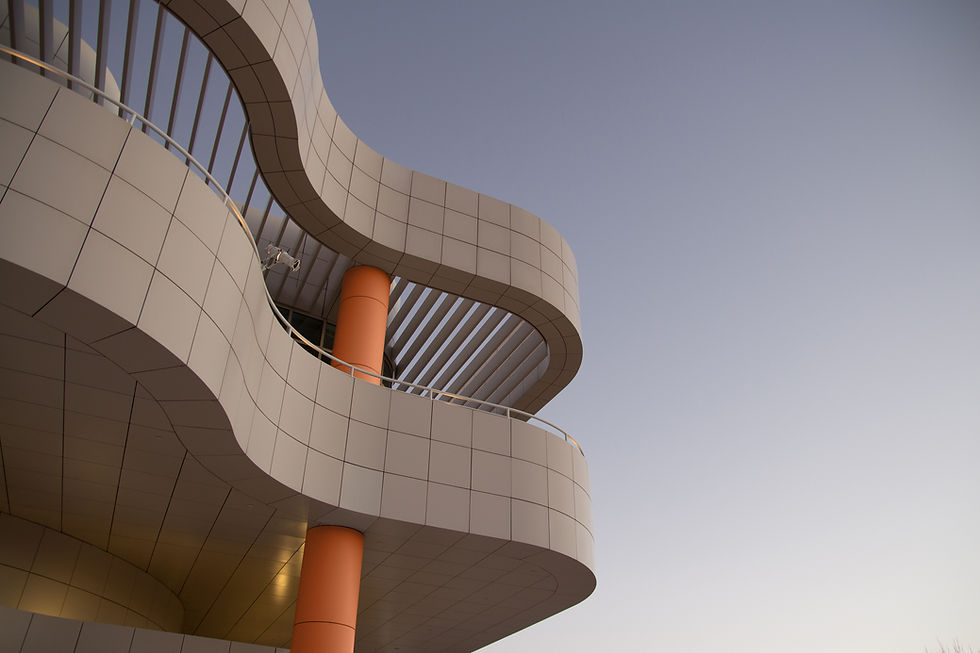
AI Policy Series 1: Drafting Your Comprehensive AI Policy
Jun 20, 2024
9 min read
0
264
0
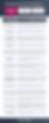
As organizations increase their adoption of AI, governance leaders are looking to put in place policies that ensure their AI deployment aligns with their organization's principles, complies with regulatory standards, and mitigates potential risks. But where to start in developing your policies can oftentimes be overwhelming.
Let’s start with some important context. AI Policies break down into 3 categories: 1) Comprehensive organizational AI policy that includes organizational principles, roles and processes, 2) an ‘AI use’ policy that outlines what kinds of tools and use cases are allowed and what precautions employees must take, and 3) a public facing AI policy that outlines core ethical principles the organization takes and their stance on key AI policy stances. Some organizations maintain a separate policy regarding 3rd party AI use, although this may be a component of other policies, or simply reflected in vendor contracts. Each policy can serve a different purpose and the combination of all 3 ensures organizations are aligned against standards like ISO 42001 and the NIST AI Risk Management Framework.
This blog post is the first in a series that explores the critical factors and decision points organizations must address when establishing their three AI policy categories outlined above. In this post, we take a deep dive into drafting a comprehensive AI policy, outlining 14 key considerations and discussing the tradeoffs you need to consider.
This blog post is not intended to provide you with an exhaustive list of considerations for organization’s AI policy. Each organization will have different needs and considerations, which must be discussed by the appropriate decision-makers. Our suggestions should be read as a starting point and not be construed as offering advice on any regulatory compliance obligations that may be applicable to your organization. Please seek advice from an attorney for further guidance on your organization’s legal obligations.
1) Determine AI Governance Owners
While it seems a bit obvious, the first thing to decide is what senior management role is responsible for determining the organization’s policy, as well as which other roles need to review and approve the policies. Many then may collect feedback from a wide range of roles, a single person, or small group, needs to be in charge of understanding policy tradeoffs, collecting input, and creating the first draft. There are a variety of trade-offs when deciding who is in charge; some organizations who develop their own AI models may want a more technical persona leading the charge. In contrast, organizations with heavy regulatory scrutiny or a non-technology focused business model may opt for a chief legal/compliance officer instead. In the case where an AI governance committee has been given the task, establishing the guidelines/charter/processes of the committee may be done first.
Guiding Questions
Who gets to decide what our AI policies are?
Whose sign off do we need on the AI policy?
2) Principles, Goals, and Frameworks
Many organizations may target specific frameworks they want to adhere to, such as ISO 42001, or the NIST AI RMF. In addition, there may be specific high level principles around human oversight, AI transparency, and bias/fairness proposed by organizations like the OECD, or from policy projects like the White House AI Bill of Rights that organizations want to adopt. Alongside the ethical value statements, organizations should also identify their overall goals and intended benefits in adopting AI technologies; this may include goals such as improving internal operations or building superior products and services. The selected frameworks, principles and goals should all align with the company’s overarching values and goals.
Guiding Questions
Are there any AI related compliance frameworks your organization wants to comply with?
How much information about your AI systems will you disclose to customers or the public?
What are the overall outcomes you want from adopting AI?
3) Specific Ethical & Legal Concerns
Many organizations have sector specific regulations, or other domain specific concerns that intersect with their AI usage. For example, the healthcare sector has specific data privacy laws and strong ethical standards to ‘do no harm’ that need to be incorporated into an organization’s AI policy, while organizations involved in the national defense space may want to ensure they only leverage models developed by specific trusted entities. Even outside of sector specific concerns, individual use cases may come with additional complexity that needs to be captured and addressed, such as use of AI for recruiting or employee performance evaluation. Other examples may include automated decision making, use by minors, or content filtering requirements
Guiding Questions
Are there any sector/domain specific rules we need to consider related to our AI use?
How will we capture any AI use case specific concerns for review?
4) Scope of Policy & AI Definitions
The definition of AI can vary widely across different industries and legal regulations. In addition, many organizations have a variety of governance structures and the policies of one entity may not always apply to subsidiaries, outsourced partners, or across business units. Establishing the scope of the AI policy across the organization, as well as what kinds of software systems fall within the scope will determine many other aspects of the policy. Leveraging the definitions of AI from established frameworks such as the NIST AI RMF or ISO 42001, or from institutions such as the OECD can be a helpful way to standardize definitions and align to regulatory expectations.
Guiding Questions
Where do you draw the line between software, algorithms and ‘artificial intelligence’?
Do one-off projects or analyses leveraging machine learning fall within the scope of the policy?
Which business units, or other subsidiaries fall within the scope of the policy?
5) AI Development Policies
For organizations that plan on developing their own AI models leveraging their own datasets, the AI policy should outline this process. Even organizations who may not be building their own models should consider a standardized process for building new AI use cases or applications using AI driven tools. This section should include any expectations for selecting datasets and models, model quality testing, bias and fairness measurement, performance monitoring, and the process for deciding whether a model is appropriate to deploy. Some of these will apply even if organizations just use off-the-shelf AI systems they run themselves, or if the organization integrates an AI system with their own data sources. This policy may also mandate any specific tools, platforms or applications that the organization is allowed to use to build new use cases.
Guiding Questions
What is the process for deciding whether a model can/should be released?
Which teams are responsible for testing a model for accuracy, and performance?
Under what circumstances may an AI model be retired or decommissioned?
6) Risk Tolerance & Management
Organizations should align on their AI risk management process, and level of risk tolerance for AI systems, especially state-of-the-art systems. For example, some organizations are willing to assume the risk of temporary reputational harm in exchange for accelerated AI innovation, while others are not. The risk tolerance may differ between different business units, or teams involved. In addition to some principles around risk tolerance, the policy should also outline how AI risks will be identified, tracked, and reviewed, whether there are any prohibited AI uses, and under what conditions an AI system will be decommissioned.
Guiding Questions
Are we willing to use the newest AI technology, or do we want to wait until the technology matures more?
Will we follow a ‘whitelist’ (only specific uses allowed) or ‘blacklist’ (most uses allowed except for specifically prohibited ones) approach to AI use cases?
Who gets to decide if an AI use case is ‘too risky’ for the organization?
How will we perform risk assessments on AI use cases, models, and vendors?
7) Internal Stakeholders
AI governance often involves a cross-functional team including technical, subject matter, and legal experts as well as others. Identifying which teams or business units need to be involved, and what their roles will be in governance should be encoded in the policy. Many organizations at a minimum involved key technical, legal, and business unit leadership, but larger organizations may also involved formal compliance, privacy, procurement, or risk departments. Some organizations have adopted a highly decentralized or distributed structure with smaller decision making committees throughout the organization, then use a centralized committee as an escalation point, and center of excellence.
Guiding Questions
Who is in charge of reviewing the technical aspects of an AI system?
Who is in charge of reviewing any legal issues associated with AI use?
Do other teams need to be involved with assessing AI risks?
8) External Stakeholders
In addition to internal stakeholders, organizations should also identify who the relevant external stakeholders may be. External stakeholders fall into 2 categories: those directly impacted from an organization’s AI use, for example the users of an AI product, and the indirectly impacted stakeholders such as anyone whose data is used to train an AI model. Larger organizations may also have broader community stakeholders, or face a heightened degree of regulatory scrutiny and want to identify a broad set of indirectly impacted stakeholders. These stakeholder groups are required for conducting regulation-mandated impact assessments.
Guiding Questions
Are there sector specific stakeholders we need to consider?
What groups may be impacted by failures in our AI systems?
How do we plan to get input and feedback from stakeholders?
9) AI Governance, Cybersecurity, and Data Privacy
Many organizations already have to comply with established cybersecurity and data privacy regulations, and AI intersects heavily with these areas, while incorporating additional new concerns. Organizations may consider combining the requirements, processes or governance structures used for compliance with these other regulations. For example, an organization should identify when an AI system may have specific data privacy or cybersecurity requirements. Organizations should identify any opportunities to combine oversight functions and ensure the AI policy implementation doesn’t conflict with other policies, and is as efficient as possible.
Guiding Questions
Does our organization have heavy obligations under data privacy laws such as GDPR?
Does our organization have a high level of cybersecurity requirements?
Are there any ways we want to unify our compliance efforts for data privacy, cybersecurity and AI?
How will cybersecurity, data privacy, and AI governance efforts be coordinated?
10) Monitoring User Feedback
Organizations should clearly outline how they will collect and document feedback about AI systems they build or deploy. This may be as simple as creating a form to fill out with potential errors about a system, or it could be more complex with formal whistleblowing policies. For organizations providing AI powered products or services, the policy may extend to how customers/users should be reporting feedback and how their feedback will be assessed. The organization should also set an expected review cadence, or review their service level agreements (SLAs) with their vendors or third parties to understand their responsiveness obligations forAI issues.
Guiding Questions
How can internal users submit feedback about an AI tool or use case?
Which teams are responsible for reviewing this feedback?
11) Transparency & Disclosure
Transparency and disclosure in AI systems may be a component of an organization’s ethical principles. However, specific regulations may also require additional disclosures. The policy should consider both transparency standards for internal AI use, as well as transparency to any users of an AI product/service. Some organizations may want to only supply information under a confidentiality agreement, while others may want to try and proactively build trust and prioritize higher transparency on their AI use. The organizational policy should outline these principles.
Guiding Questions
Will you disclose to employees when AI is being used as part of an internal operational process?
What information about your organization’s use of AI will you share publicly vs under a non-disclosure agreement?
12) AI Competency Planning
AI is a highly technical and fast moving technology. This can increase the difficulties in understanding and managing the potential risks of the systems that the organization may deploy. Organizations should establish clear criteria for hiring technical experts that will build or deploy AI systems. In addition, there should be established baseline policies and procedures to train the existing workforce on how AI systems are utilized throughout the organization and what legal obligations exist when interacting these systems. Workforce training should be tailored appropriately to roles and responsibilities. Organizations should also ensure that their policies and procedures address training for new workforce hires, as well as recurring training opportunities for the existing workforce.
Guiding Questions
How will the organization ensure it has the necessary technical expertise to develop or deploy AI?
Are there any trainings/courses your organization wants to mandate for specific AI related roles?
13) Vendor Management
AI has a very complex supply chain with both upstream vendors making many AI model design decisions that may impart risk. For example, the model creator may have used copyrighted information to train their model without proper disclosures. Similarly, there may be ‘downstream’ vendors who may be training models on an organization’s sensitive data. An organization’s policy should at least outline how they will try to capture the vendor/third party risk such as sending vendors due diligence questionnaires or mandating vendors comply with AI governance frameworks like ISO 42001 with proof of a third party audit report. The policy should indicate that vendor or third-party reviews for specific AI use cases or applications will be evaluated on a case-by-case basis to assess the risk and benefits to the organization.
Guiding Questions
How will your organization assess the risks associated with different models you buy/deploy from vendors?
How will your organization assess the AI policies for other vendors?
Who is responsible for reviewing these reviews?
14) Governance Tooling & Implementation
AI governance is quickly becoming complex as customer due diligence requests, and regulatory requirements have grown even while organizations have rolled out dozens of new AI use cases and tools. Assessing the benefits and risks of any particular AI use case or system can take time, and scaling the ability for teams to review these will have a huge impact on how fast an organization innovates. An organizational policy therefore may point to specific tools, workflows, or other strategies to implement and maintain their AI governance function.
Guiding Questions
Where will your organization maintain an AI inventory (AI models, vendors, use cases, etc)?
How can someone submit a new AI tool/use case for approval?
–
Even with this guidance on key factors and decision-points, developing your organization’s AI Policy can be overwhelming. Trustible’s Responsible AI Governance Platform helps organizations develop their AI policies by leveraging a library of templates, guiding questions, and analysis tools to ensure their policy is aligned with their values, compliant with global regulations, and equipped to manage risks.
To learn more about how Trustible can enable your organization to develop and implement a robust AI governance program, contact us here.