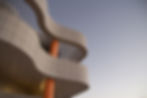
Navigating AI Vendor Risk: 10 Questions for your Vendor Due Diligence Process
Jan 2, 2024
4 min read
0
693
0

AI is everywhere, but the race to add AI from vendors has embedded unknown risks into your supply chain. Knowing what type of AI your suppliers use is difficult enough, let alone knowing how to ensure your due diligence adequately addresses the unique risks it may pose. Yet, customers and regulators are increasingly probing into how and where AI is being used throughout organizations’ supply chains. Understanding how to question your suppliers on their AI use can be a daunting task, even for the most sophisticated organizations.
This practical guide focuses on evaluating a vendor's AI application and provides some initial questions to consider as part of your due diligence process. The answers to these questions will help provide critical information to supplement traditional vendor evaluations, which typically focus on either the vendor’s organization or technical AI model performance. The key takeaway for this guide is to focus your due diligence questions on the mechanics of the AI model’s underlying technology, as well as the sustainability of their performance over time and the management of any associated risks.
Questions to Ask Your AI Vendors... and Why
CORE TECHNOLOGY
Question 1: What type of AI model does your system use and is it explainable?
Why ask this question? First, it is critical to understand what AI model type is being used given the wide array of existing AI models. It is important to know that each AI model comes with different trade-offs between their speed, complexity, and how they generate outputs. Second, it is vital to know whether the AI model being used is ‘explainable.’ These types of AI model architectures allow users to understand how AI model outputs were generated, while others are ‘black boxes.’ Specific AI use cases may require an ‘explainable’ AI model,while others may not.
Question 2: What is the intended use of your system?
Why ask this question? The AI system(s) being used may have been developed for a specific purpose, while others may be ‘general purpose’. While some AI systems can be creatively repurposed, this may come with some risks and limitations. Understanding what an AI system was originally meant to do can help identify if it is ‘fit for purpose’ to complete a specific task.
TRAINING DATA
Question 3: What is the source of your training data for the AI model?
Why ask this question? One of the largest limitations for an AI model is the sources of original training data. This is because every data source will come with its own selective effects based on the population that generated the data. Moreover, there are no well established ‘universal’ datasets that represent, among other things, every culture, demographic group, and language. There may also be ethical or legal implications based on the training data. For example, using copyrighted data for AI model training could come with limitations on its use in specific jurisdictions. Additionally, it may be critical that the training data was collected from a certain population that an AI system is serving.
Question 4: Will inputs to the system be used as training data?
Why ask this question? Some laws require that AI model inputs be preserved for a period of time to monitor the AI model’s performance. However, there are instances where AI model inputs may be used as additional training data. This can be an issue if certain information (e.g., personally identifying information or trade secrets) is used as part of the AI model inputs.
PERFORMANCE
Question 5: How was the AI model tested, evaluated, validated, and verified?
Why ask this question? After an AI model is trained, it typically goes through several quality assurance steps (e.g., automated evaluation against test data or community benchmarks). Additionally, the AI model may be subject to human validation, such as review by AI model validation teams or third party ‘red teaming’ exercises. Knowing what evaluations were done, as well as how an AI model performed on them, can help set reasonable expectations for AI model performance.
Question 6: What are known limitations of the system?
Why ask this question? AI models have specific limitations due to their original training data, AI model design decisions, or update frequency. These AI model limitations should be clearly documented. Additionally, your organization should consider the interplay between the existing AI model limitations and those limitations your organization may want to impose on them.
RISK MANAGEMENT
Question 7: What are your organization’s AI policies?
Why ask this question? AI governance is not limited to AI models, but rather it requires buy-in from every level of the organization. This includes documenting and implementing policies to address an AI system’s trustworthiness, transparency, and responsible design. When considering vendors, it is important to consider what policies and procedures are in place to oversee AI systems throughout their life cycles. Vendors should also be able to demonstrate compliance with applicable AI governance regulations.
Question 8: How have you operationalized your AI policies?
Why ask this question? AI policies help describe what an organization intends to do when developing and deploying their AI solutions. However, how it operationalizes those policies is equally as important.
Question 9: How do you document your AI systems?
Why ask this question? Improving transparency within the AI supply chain requires that organizations have clear documentation for their AI systems. Documentation should cover both technical and non-technical aspects throughout the system’s life cycle. It should be traceable, with clear individuals or teams responsible for each section of documentation. Recording and retaining this information is especially important for complying with regulations that require transparency from third parties.
ETHICAL CONSIDERATIONS
Question 10: What bias and fairness considerations went into your AI model?
Why ask this question? There are many ways to measure potential bias and fairness in an AI system. It is not enough to say an AI model is ‘fair’ and ‘unbiased’ without providing what metrics were used to determine that. Having a quantitative understanding of an existing AI system’s bias can help with subsequent bias testing.
Conclusion
When selecting the right AI vendor, you will need to look beyond their technical capabilities and cost. Instead, you must also prioritize understanding how a supplier's AI system will interact with your organization’s operations. By using these questions as a starting point in your due diligence process, you are doing more than simply evaluating a product; you are ensuring its fulfilling your organization’s strategic, operational, and ethical goals.