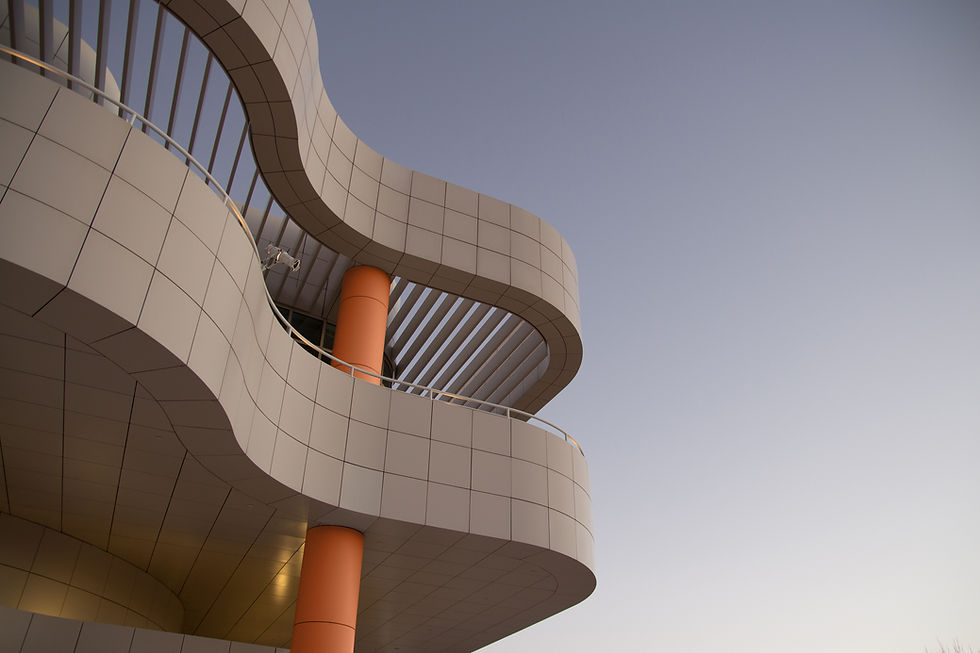

In this blog post, we will discuss the difference between the concepts of ‘internal’ monitoring, and ‘external’ monitoring and how both are required for several emerging AI governance frameworks.
A common compliance requirement across many AI regulations and voluntary AI standards is the need to monitor AI systems. However, the term ‘monitoring’ can be overloaded. Moreover, many technical teams misinterpret the regulators’ intention and believe that the requirement is simply to track the logs and input/output metrics of an AI model. While this type of monitoring is undoubtedly beneficial and necessary, there are other forms of monitoring that are required.
Internal Monitoring
When many technical personas hear the term ‘monitoring’, they often think of internal monitoring of the AI system. This type of monitoring is all about technical teams looking at the actual model that is running and the infrastructure that supports it, as well as responding to any technical incidents that may be detected by logs or application firewalls. Internal monitoring is already done on top of non-AI software systems to ensure that an application is always running and performing as expected. Internal monitoring is also frequently done for cybersecurity purposes to detect any potential adversarial cyber attacks against a specific application. Specific elements of internal monitoring may include:
Benchmark Monitoring: running various accuracy, bias, and safety benchmarks against the model on a regular basis, especially as new versions are developed and released.
Application Logging: capturing the key inputs and outputs of an AI application, and ensuring that access to those sensitive logs is well governed.
Infrastructure Monitoring: ensuring that the infrastructure supporting the AI application is meeting expected latency, cost, and throughput metrics and monitoring for potential infrastructure cyberattacks.
Performance Uptime: ensuring that the system is appropriately available according to any set SLAs, and that automatically detect error rates are within an acceptable level.
Internal Access Control: ensuring that access to the system is appropriately governed, and that unapproved, or unauthorized access is quickly detected and alerted on.
Internal Quality Reviews: implementing a quality assurance process to ensure that changes to the AI system will meet expected performance and safety goals.
Generally, these elements are all directly accessible and observable by the technical teams supporting the system. However, AI systems have additional complications beyond normal software issues, which limits what internal monitoring can cover. For example, measuring for ‘model drift’ (departure from the model’s expected performance) can be difficult to do simply by looking at the logs because the ‘correct’ result may not be known for novel model outputs. Similarly, an AI system can actually work as intended but still cause real world harm due to poor design decisions, unknown system biases, or poor user operation. These challenges are not unknown to regulators, especially those who oversee the safety of physical goods. Therefore, some AI laws like the EU AI Act take a ‘product safety’ approach and require some form of external monitoring of AI systems.
External Monitoring
External monitoring means diligently collecting, and responding to, information on how a system performs in the real world. This includes making it clear how system users can report potential issues, having processes in place for assessing these reports, and then ensuring that verified issues are addressed in a timely manner with new mitigation efforts. External monitoring already occurs in sectors such as healthcare because it is the main way that drug manufacturers can get information about harmful or unintended side effects. Some regulators want to go as far as requiring AI system developers to have processes in place for decommissioning a harmful AI system if the reported incidents exceed predetermined thresholds. This form of monitoring includes elements such as:
Incident Reporting: the process of collecting potential harms, policy violations, or misuse from an existing model, triaging them to establish validity and intensity, followed by a potential report to regulators about the issue.
User Feedback: users may seek to submit feedback about an AI system, even if it didn’t cause an incident. This feedback should be documented in case it constitutes specific risks, or is evidence of the AI system failing to meet its goals.
Business Value Review: a review of how well the AI system is meeting its intended purposes and business goals. This is to ensure that AI is only used in cases where it adds clear value, but this often requires engaging the actual end users.
Misuse Detection: a process about ensuring that an AI system is being used within its own policy for use. This may include ensuring that certain legal or ethical restrictions are being obeyed by the end users.
Scope Drift Monitoring: an AI system may initially be deployed with a specific scope or use case in mind, but this scope could increase or drift over time as new users adopt the system, and find new ways to leverage it.
Vendor Monitoring: many organizations are now relying on third-parties for the AI systems, therefore conducting regular assessments or audits on those vendors, and monitoring for potential incidents or breach by them becomes essential.
Many of the external monitoring requirements take into account the specific use case of the AI system, not simply the model. In the era of general purpose AI systems, monitoring systems from this viewpoint is essential to ensure that AI is being used in a responsible manner by the teams deploying them. Taking into account the use case is also essential because regulatory frameworks set requirements based on how AI systems are being used.
Monitoring Requirements in Key AI Regulations and Frameworks
To get a sense of how monitoring is discussed in regulatory frameworks, we can look at the language used in a few of the most impactful AI regulations from the past few years.
NIST
Both internal and external monitoring is covered by the NIST AI RMF. While many sections have implicit monitoring requirements in order to implement, sections are exclusively focused monitoring including:
GOVERN 1.5 - Ongoing monitoring and periodic review of the risk management process and its outcomes are planned, organizational roles and responsibilities are clearly defined, including determining the frequency of periodic review. (External monitoring)
GOVERN 5.1 - Organizational policies and practices are in place to collect, consider, prioritize, and integrate feedback from those external to the team that developed or deployed the AI system regarding the potential individual and societal impacts related to AI risks. (External monitoring)MANAGE 4.3 - Incidents and errors are communicated to relevant AI actors including affected communities. Processes for tracking, responding to, and recovering from incidents and errors are followed and documented. (External monitoring)
MEASURE 2.4 - The functionality and behavior of the AI system and its components – as identified in the MAP function – are monitored when in production. (Internal monitoring)
MEASURE 3.1 - Approaches, personnel, and documentation are in place to regularly identify and track existing, unanticipated, and emergent AI risks based on factors such as intended and actual performance in deployed contexts. (Internal & External monitoring)
ISO 42001
ISO 42001 details the requirements of an organizational AI risk management system, which includes monitoring in several sections. A key part of ISO 42001 is the need to constantly be evaluating whether risk mitigation efforts are sufficient for a given use case. Collecting user feedback from external monitoring is an essential component of that. Additionally, Section 9 discusses general Performance Evaluation steps organizations should adopt, including internal technical monitoring requirements, and the internal audit and improvement processes organizations should adopt.
EU AI Act
The EU AI Act has several internal monitoring requirements for high risk AI systems implied in Articles 9 (Risk Management), 10 (Data Governance), 12 (Record Keeping), and 17 (Quality Management System). Although specific details on these articles will be further clarified by upcoming standards by the EU standards body (CENELEC).
In addition, the AI Act has very specific external monitoring requirements under Article 72 (Post-market monitoring by providers and post-market monitoring plan for high-risk AI systems). This section outlines all of the requirements that AI providers need to implement to ensure that system users are able to provide feedback that is promptly reviewed and acted upon.
Colorado
The Colorado Consumer Protections for Artificial Intelligence bill (24-205) imposes risk management requirements for deployers of high risk AI systems. A core part of those risk management requirements is documented a ‘description of the post market monitoring and user safeguards…established by the deployer’. This language mirrors the EU AI Act and requires an external monitoring system be put in place.
Singapore
Singapore’s Model AI Governance Framework includes oversight provisions for organizations to clearly define roles and responsibilities to monitor deployed AI models. The framework also provides three broad approaches to classifying various degrees of human-oversight in the AI decision-making process. These approaches include human-in-the-loop, human-out-of-the-loop, and Human-over-the-loop.
How Trustible Helps
Trustible’s AI governance platform helps organizations with all the requirements for external monitoring, and integrates with best in class MLOps solutions and cloud platforms in order to verify that appropriate internal monitoring is in place.
Trustible has out-of-the-box guided workflows for responding to AI incidents, conducting business reviews, and tracking proposed scope changes. These workflows help organizations maintain paper trails of their decisions about AI systems and capture the inputs of their AI governance work. Meanwhile, Trustible’s proprietary regulatory and risk insights also help ensure that organizations are alerted to new regulatory guidance, potential AI risks, and recommended mitigation best practices. Trustible’s AI inventory also allows organizations to systematically track feedback they receive from users about their AI systems, log any potential incidents, and build an audit trail demonstrating compliance with the post-market monitoring requirements of regulations like the EU AI Act.
Conclusion
Overall, AI monitoring encompasses internal and external aspects, each playing a crucial role in ensuring AI systems' safety, effectiveness, and compliance with emerging AI governance frameworks. Internal monitoring focuses on the technical health and performance of the AI system, while external monitoring involves gathering and responding to real-world feedback and usage data. Key AI regulations and frameworks, such as the NIST AI RMF, ISO 42001, the EU AI Act, Colorado's AI bill, and Singapore’s Model AI Governance Framework emphasize the importance of both internal and external monitoring. Responsible AI deployment and regulatory compliance require implementing both forms of monitoring.