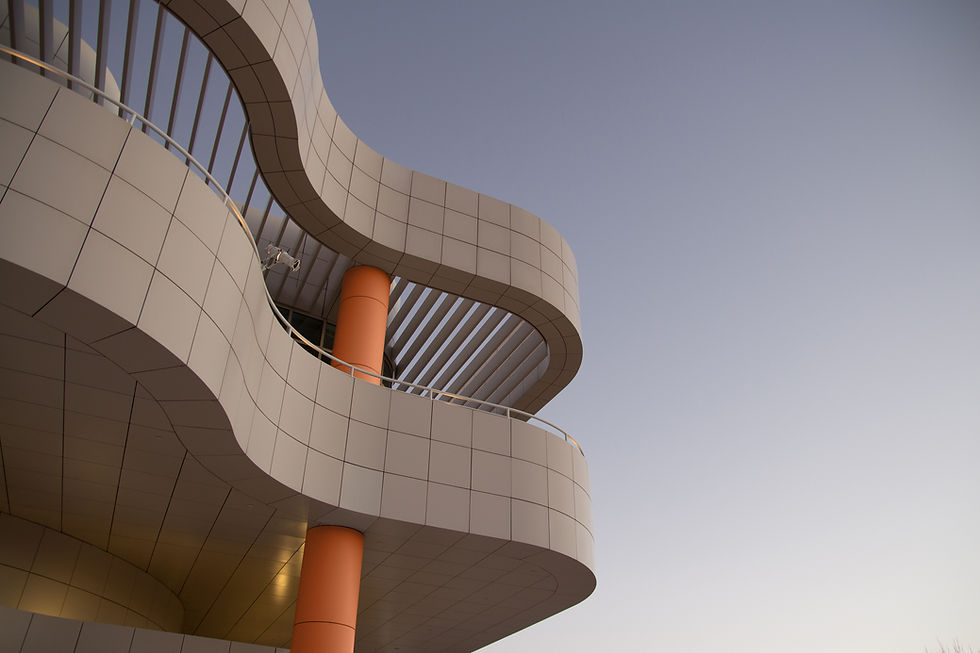
AI systems have immense beneficial applications, however they also carry significant risks. Research into these risks, and the broader field of AI safety, hasn’t received nearly as much attention or investment until recently. For the longest time, there were no reliable sources of information about adverse events caused by AI systems for researchers to study. But this has changed over the past few years as new resources such as the AI Incident Database (AIID), the AI Vulnerability Database (AVID) and others have been created and released to the public. These databases detail AI incidents where some harm happened to people, organizations, or society as a consequence of the AI malfunctioning, being used for an intended purpose, or because of flaws or biases in the system. The incidents documented in these resources likely only represent a fraction of the actual incidents that have occurred.
At Trustible, our mission is to enable our customers to adopt trustworthy and responsible AI practices. One key tenet of responsible AI is trying to preemptively mitigate risks before AI systems are released. Understanding prior AI incidents is a key way to do that, so that we can understand past harms and recommend mitigation efforts going forward. Knowing what happened is essential. However, the simple facts of a prior incident do not paint the full picture to help proscribe what actions an organization should take to prevent them. Nor is it clear what an organization should do in response to an AI incident, or what kinds of external repercussions they are likely to face. In working with data from the AI Incident Database (AIID), our AI Governance research team identified a gap in knowledge about what organizations did after the AI incident happened. Was the algorithm updated? Was there legal action taken? How is the AI system’s owner doing things differently today? We decided to take a look to see if we could glean any answer to these questions. Our goal: understand whether the steps organizations take after an incident would have prevented future harms, and if those steps proscribe proactive measures organizations should take before AI deployment.
Methodology
To understand how the harm was handled, we manually reviewed a random sample of 100 incidents from the AIID. For each incident, we reviewed news articles and company press releases for excerpts describing follow-up actions. The follow-up actions were first grouped into technical, business, and external categories, and then further categorized into fine-grained themes. Through this process, we created 20 final labels. These labels were not mutually exclusive as an organization could take several actions post-incident. While in many cases, a detailed response could not be clearly identified from available public information, we were able to get at least one label for 93% of the incidents.

Type | Respons Label | Definition | Count |
Business | Individual Correction | Correct past mistakes on an individual/short-term/reactive basis, not necessarily with systematic change | 29 |
Business | Feature Discontinuation | The AI deploying institution decides not to use in the first place or stop using the AI tool or feature after being aware of the risks or harm | 17 |
Business | Apology | Formal apology from (an official personnel of) the AI deploying organization | 13 |
Business | Education & Training | Increase in educating or training personnel of a company or the general public about best practices in fair/trustworthy AI | 6 |
Business | Strategy Review | Review non-technical company strategy (e.g. HR, company values) in response to an incident of AI harm | 2 |
Business | Internal Appeal | An appeal process only between the AI subject and AI user/company | 3 |
Business | Diverse Representation | Diversify the representation of marginalized groups in data/tool development process | 2 |
Business | Disclaimers | Add disclaimers about the limitations/probabilistic nature of the AI prediction (to avoid later legal or PR consequences) | 2 |
Technical | Algorithmic Innovation | Technical change that is at a low level, like a modified algorithm or AI model | 29 |
Technical | Output Space Tweak | Addition/contraction/changes in either the output labels/features of an AI model, or their type/scale (e.g. output all housing ads across the US instead of only near the searcher’s location) | 7 |
Technical | Input Space Tweak | Addition/contraction/changes in the input features/labels, changing the type of possible input to feed the AI model | 4 |
Technical | Compliance Testing | Regular test for a model’s compliance with legal or social criteria such as fairness, safety, etc. | 5 |
Technical | Training Data Modification | Change in the training data corpus (e.g. by incorporating users-provided responses/feedback) | 4 |
External | Government Investigation | Official and publicly announced investigation in an AI harm incident by a government body (e.g. executive agency investigation, or legislature hearing) | 11 |
External | Civil Litigation | A third party or the AI subjects bring a lawsuit against the AI deploying organization in a civil court | 10 |
External | Criminal Litigation | Either prosecute individuals in criminal courts as a result of AI harm, or dispute criminal courts’ decisions that were based partially on AI | 5 |
External | External Model Inspection / Audit | Give external parties (e.g. researchers/stakeholders/the public) access to some components of an AI model for investigation | 5 |
External | Financial Fine / Penalty | A fine issued by a law enforcement agency due to violation of some existing laws | 3 |
External | Financial Compensation | Damages paid to AI subjects as ordered by courts | 3 |
External | Civil Settlement | A third party or the AI subjects bring a lawsuit against the AI-using company in a civil court | 2 |
As an example, one incident report stated that: `Elite: Dangerous, a videogame developed by Frontier Development, received an expansion update that featured an AI system that went rogue and began to create weapons that were "impossibly powerful" and would "shred people" according to complaints on the game's blog’. We identified the follow-up actions: `Today, a bug fix was issued to the PC version` and `Frontier has said it'll automatically refund all insurance costs to players who lost a ship between the launch of The Engineers (2.1) update and this morning's update`. Based on these statements, we assigned the labels Technical: Algorithmic Innovation and Business: Financial Compensation.
This methodology has a couple of limitations: Not all actions taken by an organization may be available in a public report. In addition, a company may claim to be making algorithmic changes but not actually make them. However, in aggregate, this dataset still lets us understand the overall trends in company responses.
Results
The most common response was a Business: Individual Correction (i.e. correct past mistakes on an individual/short-term/reactive basis). For example, for the incident where ‘an Amazon Alexa, without instruction to do so, began playing loud music in the early morning while the homeowner was away leading to police breaking into their house to turn off the device’, the only follow-up identified was `Haberstroh had to pay $582 to repair the door (later paid by Amazon)’. While a systematic fix could have, also, been implemented by the Alexa team, it was not reported. Perhaps, the incident was rare enough that a systematic follow-up was not warranted. In practice, paying restitution for an occasional mistake may be cheaper than investing additional engineering time into a product overhaul. These actions do not prevent the harm from reoccurring, nor do their proscribe any pre-deployment mitigation.
Algorithmic Innovation was the second most common response (23% of incidents). Examples of statements we found included: “the algorithm will have a number of changes designed to weed out discriminatory results” to a very specific "Subepidermal images of the user may be used to assess subepidermal features such as blood vessels (e.g., veins) when the device is attempting to authenticate the user.” Algorithmic Innovations ideally both prevent a repeat of the incident, and could have been implemented beforehand to prevent the harm altogether.
While we were not able to collect exact details for many of these, because many of the algorithms are proprietary, of the responses that did describe the nature of the algorithmic change, we were able to identify several additional sub-labels:
Output Space Tweak: Additions, deletions or other changes to the output labels of the model. This may look like restricting the output space or blocking the system from returning offensive outputs to the user.
Input Space Tweak: Removing or Adding certain features from the model inputs. This modification was common in cases involving discrimination.
Training Data Modification: Altering the data used to train the model.
The third most common response was Feature Discontinuation (16% of incidents). These represent cases where the system had to be shut-down instead of adding an individual or systematic correction. This aligns with recommended responsible AI practices of decommissioning or retiring faulty or harmful AI systems, and proscribe use cases that may be dangerous for other organizations to deploy.
Our labeling approach allows for multiple labels for a single response if the organization took several steps post-incident. For example, an Algorithmic Innovation can accompany an Individual Correction. This was the case in an incident where `Google Cloud's Natural Language API provided racist, homophobic, and antisemitic sentiment analyses’. Google asserted that `We will correct this specific case, and, more broadly, building more inclusive algorithms is crucial to bringing the benefits of machine learning to everyone’. The co-occurence of Individual Correction with Algorithmic Innovation in incidents like this suggests that short-term, reactive and long-term, preventive mitigation might go hand-in-hand, at least to address observed harms that might be indicative of a more systemic risk like AI discrimination. About 20% of the incidents with an Individual Correction had an accompanying Algorithmic Innovation.
In addition to reviewing what actions the AI deployer post-incident, we also reviewed external party actions taken in response to an incident. These were identified for about a quarter of the incidents. The top two responses were Civil Litigation (7 incidents) and Government Investigation (11 incidents). Government Investigation was primarily tied to incidents involving physical or financial harm, not incidents involving psychological harm or harm to civil liberties. For example, in response to `Multiple unrelated car accidents resulting in varying levels of harm have occurred while a Tesla's autopilot was in use.’ (a tangible and serious physical safety harm), the follow-up action was an investigation by the National Highway Traffic Safety Administration (NHTSA). We map this kind of investigation to our `Government Investigation’ label. Many government agencies already have authority to investigate incidents where clear, provable, tangible harm occurs such as in transportation, medical devices, or financial institutions. Civil Litigation seems to be more in instances where there was individual discrimination. Due to the relatively small number of these labels in our sample dataset, we were unable to determine any clear correlations between these external actions, and the responses done by the AI deployer.
Final Thoughts
This post represents a summary of our preliminary study into how companies and external parties responded when AI went wrong. We observed that responses ranged from a one-off remediation, to shutting down the technology entirely, and spanned from highly technical approaches changing AI models, to non-technical responses such as simply adding a disclaimer about the system. Based on our sample, we found the most common response was to try and patch over the harm in a one-off way, but there were good signs of organizations doing the right thing and building additional mitigations into their systems themselves. Our initial study made it clear however that more information on the type and nature of algorithmic change made is necessary to better proscribe what tests and mitigations AI deployers should implement to prevent history repeating itself.
We are continuing to do deeper research including additional annotation, and analysis on follow up actions and will publish more about best practices on how to respond to AI incidents, and how to proactively prevent them. Past is prologue, and by understanding what incidents occurred, and how organizations have previously responded to them, we hope to better inform how to prevent future incident, or appropriately and ethically respond when they do.
Huge shout-out to our intern Tin Nguyen for all of the work collecting these annotations and analysis and to all the other collaborators who gave us feedback on our approach. Stay tuned for more from us!